RECENT RESEARCH
My recent and current research agenda focuses on the modeling and prediction of financial asset returns. In particular, my team and I develop and study multivariate stochastic processes suitable for complex data arising from a variety of financial instruments. By calibrating these constructs to real data with sophisticated statistical methodologies, we can make accurate predictions of risk and return.
This, in turn, leads to algorithms for improved asset allocation. We derive optimal investment strategies and portfolio weights, through time, for a given universe of risky assets, such as stocks, bonds, currencies, options, crypto-currencies, and commodities, accounting also for transaction costs and illiquidity issues.
​
To get an idea of the improvements we obtain, Graphic 1 shows the performance of some of our models when applied to the stocks of the DJIA (top lines; total return over time) versus existing investment strategies (bottom lines) such as equally weighted (1/N), Markowitz, and traditional GARCH.
​
The various methods I work on can be divided into five categories (see Graphic 2). The top four are based on multivariate constructs, and all support large-dimensional asset universes, despite the proliferation of parameters and the "curse of dimensionality". The fifth method is for multivariate portfolio allocation, but makes use of only simple, univariate models that have been augmented with extremely fast statistical and numerical approximation procedures to allow it to work.

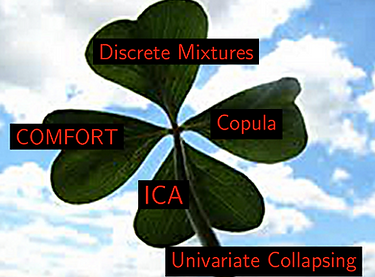
Graphic 1: Performance of competing methods
Graphic 2: Five different categories of methods
The academic papers associated with these five methods are listed below in their respective categories.
The "COMFORT" methodology (acronym for COmmon Market Factor nOn-Gaussian ReTurn Model) is arguably the most interesting one from a purely theoretical / academic viewpoint, and is among the best performers. The third graphic shows a diagram indicating the core COMFORT model and the numerous extensions we are working on.
A famous quote (often attributed to Kong Tzu, better known as Confucius) is "A scholar who cherishes the love
of comfort is not fit to be deemed a scholar." There is much truth to this, as we need to relentlessly study and learn new things,
both for the sake of learning, and in order to generate worthwhile new research --- which is often very challenging and time-consuming. However, in our case, "COMFORT" is a core part of our academic efforts! We knew this is a promising and fortuitous approach---the fortune cookie we received, pictured on the right ("You will always be surrounded by comfort") is real.
A complete list of my published research work, along with citation information, can be found on my Google Scholar page.
Discrete Mixtures
2025
Low Dimensional Home Bias Portfolio Optimization
S. Chitsiripanich, M. Paolella, and P. Polak
2017
Robust Normal Mixtures for Financial Portfolio Allocation
M. Gambacciani and M. Paolella
Econometrics and Statistics, 3, pp. 91-111
2015
Multivariate Asset Return Prediction with Mixture Models
M. Paolella
European Journal of Finance, 21(13-14), pp. 1214-1252
2013
Stable Mixture GARCH Models
S. Broda, M. Haas, J. Krause, M. Paolella, and S.-C. Steude
The Journal of Econometrics, 172(2), pp. 292-306
2012
Mixture and Regime-Switching GARCH Models
M. Haas and M. Paolella
In Bauwens, L., Hafner, C., and Laurent, S., (eds.)
Handbook of Volatility Models, John Wiley & Sons
2009
Asymmetric Multivariate Normal Mixture GARCH
M. Haas, S. Mittnik, and M. Paolella
Computational Statistics and Data Analysis, 53(6), pp. 2129-2154
2008
An Econometric Analysis of Emission Trading Allowances
L. Taschini and M. Paolella
Journal of Banking and Finance, Vol. 32, pp. 2022–2032
2004
A New Approach to Markov-Switching GARCH Models
M. Haas, S. Mittnik, and M. Paolella
Journal of Financial Econometrics, 2(4), pp. 493-530
2004
Mixed Normal Conditional Heteroskedasticity
M. Haas, S. Mittnik, and M. Paolella
Journal of Financial Econometrics 2(2), pp. 211-250
Continuous Mixtures / COMFORT
2025
Risk Parity Portfolio Optimization under Heavy-Tailed Returns and Dynamic Correlations
M. Paolella, P. Polak, and P. Walker
Journal of Time Series Analysis (forthcoming)
2025
A New Class of Heterogeneous Tail Models for Financial Asset Returns
M. Paolella, P. Polak, and F. Sandmeier
2021
A Non-Elliptical Orthogonal GARCH Model for Portfolio Selection under Transaction Costs
M. Paolella, P. Polak, and P. Walker
​Journal of Banking and Finance (available online)
2019
Regime Switching Dynamic Correlations for Asymmetric and Fat-Tailed Conditional Returns
M. Paolella, P. Polak, and P. Walker
Journal of Econometrics, 213(2), pp. 493-515
2019
Heterogenous Tail Generalized COMFORT Modeling via Cholesky Decomposition
J. Naef, M. Paolella, and P. Polak
Journal of Multivariate Analysis, 172, pp. 84-106
2015
COMFORT: A Common Market Factor Non-Gaussian Returns Model
M. Paolella and P. Polak
Journal of Econometrics, 187(2), pp. 593–605
Fractional Differencing for
Portfolio Optimization
2025
Momentum Without Crashes
S. Chitsiripanich, M. Paolella, P. Polak, and P. Walker
2025
Smoothing Out Momentum and Reversal
S. Chitsiripanich, M. Paolella, P. Polak, and P. Walker
Copula
2018
COBra: Copula-Based Portfolio Optimization
M. Paolella and P. Polak
In Kreinovich, V., Sriboonchita, S., and Chakpitak, N. (eds.)
Predictive Econometrics and Big Data, Springer-Verlag, pp. 36-77
ICA
2009
CHICAGO: A Fast and Accurate Method for Portfolio Risk Calculation
S. Broda and M. Paolella
Journal of Financial Econometrics, 7(4), pp. 412-436
2015
ALRIGHT: Asymmetric LaRge-Scale (I)GARCH with Hetero-Tails
M. Paolella and P. Polak
International Review of Economics and Finance, 40, pp. 282-297
Univariate Collapsing
2017
The Univariate Collapsing Method for Portfolio Optimization
M. Paolella
Econometrics, 5(2), article 18, pp 1-33
2014
Fast Methods for Large-Scale Non-Elliptical Portfolio Optimization
M. Paolella
Annals of Financial Economics, 9(2)
Risk Calculation
2018
Approximating Expected Shortfall for Heavy-Tailed Distributions
S. Broda, J. Krause, and M. Paolella
Econometrics and Statistics, 8, pp. 184-203
2014
A Fast, Accurate Method for Value-at-Risk and Expected Shortfall
J. Krause and M. Paolella
Econometrics, 2, pp. 98-122
2013
Time-varying Mixture GARCH Models and Asymmetric Volatility
M. Haas, J. Krause, M. Paolella, S.-C. Steude
North American Journal of Economics and Finance, 26, pp. 602-623
2011
Expected Shortfall for Distributions in Finance
S. Broda and M. Paolella
In Cizek, P., Haerdle, W., and Weron, R., (eds.)
Statistical Tools for Finance and Insurance, 2nd edition, Springer Verlag
2008
Risk Prediction: A DWARF-like Approach
M. Paolella and S-C. Steude
The Journal of Risk Model Validation, 2(1), pp. 25-43
2006
Value-at-Risk Prediction: A Comparison of Alternative Strategies
K. Kuester, S. Mittnik, and M. Paolella
Journal of Financial Econometrics 4(1), pp. 53-89
2003
Prediction of Financial Downside–Risk with Heavy–Tailed Conditional Distributions
S. Mittnik and M. Paolella
In Rachev, S. T. (editor)
Handbook of Heavy Tailed Distributions in Finance, Volume 1, Chapter 9, Elsevier North–Holland
2000
Value-at-Risk Prediction: A Comparison of Alternative Strategies
K. Kuester, S. Mittnik, and M. Paolella
Journal of Financial Econometrics 4(1), pp. 53-89
2000
Conditional Density and Value-at-Risk Prediction of Asian Currency Exchange Rates
S. Mittnik and M. Paolella
Journal of Forecasting, 19, pp. 313-333
2000
Diagnosing and Treating the Fat Tails in Financial Returns Data
S. Mittnik, M. Paolella, and S. Rachev
Journal of Empirical Finance, 7
1999
A Simple Estimator for the Characteristic Exponent of the Stable Paretian Distribution
S. Mittnik and M. Paolella
Mathematical and Computer Modelling, 29
Distributional Computation and Testing
ARIMA Time Series Analysis
2016
Stable-GARCH Models for Financial Returns: Fast Estimation and Tests for Stability
M. Paolella
Econometrics, 4(2), Article 25
2016
Asymmetric Stable Paretian Distribution Testing
M. Paolella
Econometrics and Statistics, 1, pp. 19-39
2015
New Graphical Methods and Test Statistics for Testing Composite Normality
M. Paolella
Econometrics, 3(3), pp. 532-560
2017
Autoregressive Lag–Order Selection Using Conditional Saddlepoint Approximations
R. Butler and M. Paolella
Econometrics, 5(3), pp. 1-33
2009
Assessing and Improving the Performance of Nearly Efficient Unit Root Tests in Small Samples
S. Broda, K. Carstensen, and M. Paolella
Econometric Reviews, Vol. 28(5), pp. 1-27
1998
Approximate Distributions for the Various Serial Correlograms
R. Butler and M. Paolella
Bernoulli, 4(4), pp. 497-518
2009
Evaluating the Density of Ratios of Noncentral Quadratic Forms in Normal Variables
S. Broda and M. Paolella
Computational Statistics and Data Analysis, Vol. 53(4), pp. 1264-1270
2008
Uniform Saddlepoint Approximations for Ratios of Quadratic Forms
R. Butler and M. Paolella
Bernoulli, Vol. 14(1), pp. 140-154
2007
Saddlepoint Approximations for the Doubly Noncentral t Distribution
S. Broda and M. Paolella
Computational Statistics and Data Analysis, Vol. 51, pp. 2907-2918
2002
Saddlepoint Approximation and Bootstrap Inference for the Satterthwaite Class of Ratios
Journal of the American Statistical Association, 97(459), pp. 836-846
2002
Calculating the Density and Distribution Function for the Singly and Doubly Noncentral F
Statistics and Computing, 12(1), pp. 9-16
2002
Stationarity of Stable Power–GARCH Processes
S. Mittnik, M. Paolella, and S. Rachev
Journal of Econometrics, 106, pp. 97--107
